Alternating Optimization of Deep Recurrent Neural Networks for Transmitter Adaptation in UWOCs
Research | | Links:
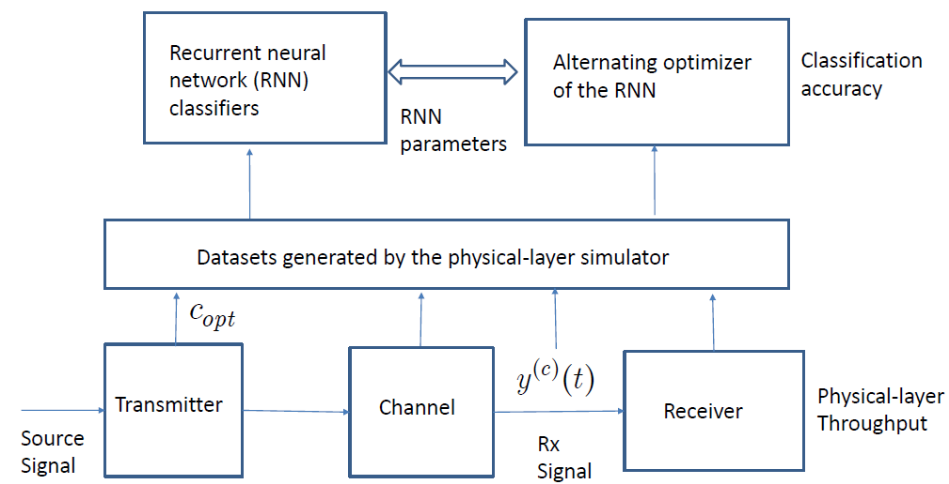
In most communication systems now-a-days, the traditional non-machine-learning (non-ML) rate adaptation approach is to perform a look-up table search based on the SNR estimates at the receiver. Albeit widely accepted and implemented in a broad range of commercial communication systems including cellular mobile networks as well as WiFi systems, the SNR-based approach has intrinsic limitations in identifying the optimal transmitter configuration. The SNR estimates at the receiver have bias and the information of the received signal waveform is lost at the SNR estimation step. These limitations put constraints on its applications in low-SNR applications. The research problem investigated in this work is the optimization of the Recurrent Neural Network (RNN) classifiers to adapt the transmitter configurations in the wireless underwater optical communications (UWOCs). The RNNs are adopted at the receiver to identify the optimal transmitter configuration based on the received signal waveform. Four types of RNNs, including Long Short-Term Memory (LSTM), Bi-directional LSTM (Bi-LSTM), Gated Recurrent Unit (GRU) and our proposed RNN named SwitchOpt RNN, are evaluated on the transmitter configuration adaptation problem in underwater optical networks. An algorithm framework to optimize the key design parameters of RNNs based on the alternating optimization is designed for the four types of RNNs evaluated, on classifying binary, three-class and six-class transmitter configurations of varying spreading factor and coding rate. The proposed SwtichOpt RNN and its alternating-optimized architecture are compared with the traditional non-machine-learning (non-ML) transmitter adaptation based on the SNR estimation.