Wireless Body Area Sensor Networks for Bio-medical Applications
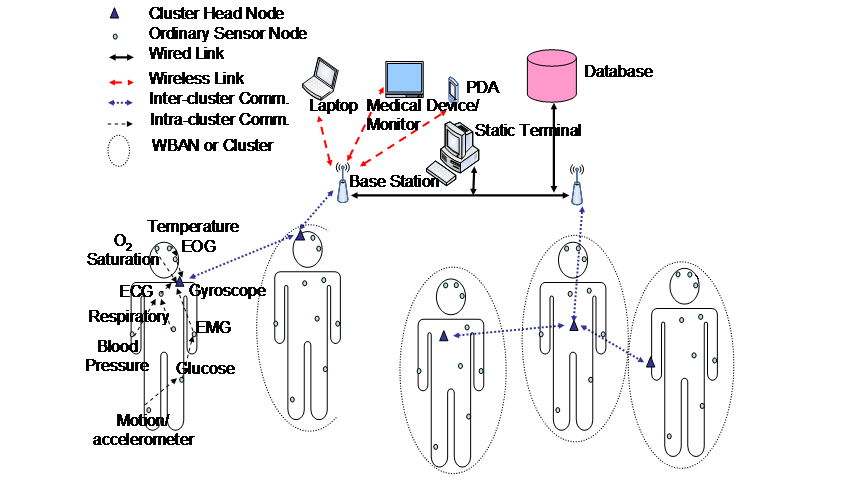
Researchers at CPS lab are investigating communication, data fusion and information processing challenges associated with Wireless Body Area Networks (WBANs) for bio-medical applications.
One of the topics in this area of research is “continuous vital monitoring for trauma triage using Wireless Sensor Networks (WSNs)”. Trauma triage is the process of classifying injured patients based on their need for or likely benefit from trauma center care. Accurate and reliable monitoring of the patients vital signs during this period is crucial for making efficient and error-free triage decisions. Current limitations of patient monitoring represent an important barrier for developing improved trauma triage methods.
The wireless sensor based system that is being developed will continuously and noninvasively monitor the patient in the pre-hospital and hospital settings (see figure). Once deployed on the patient, the system will automatically and continuously perform the three following operations: 1) record measurable patient data such as vital sign measurements (pulse, blood pressure, respiratory rate); 2) capture patient data that now relies on observation alone (e.g., level of consciousness); 3) issue alerts to the medical team in the hospital if critical deviations are observed or predicted. The data will be seamlessly and reliably transmitted to portable devices, such as PDAs, smart phones, and hand-held small computers, using wireless communications.
Another topic that is being currently studied is “real-time online correlation of human physical activities and vital signs (e., ECG)” remotely collected using a WBAN consisting of inertial sensors (accelerometers and gyroscopes) and bio-medical sonsors (ECG sensors). Online correlation of physical activities with vital signs wil have a tremendous impact on solutions for inexpensive solutions for remote diagnosis and health monitoring in the developing world. Human physical activities will be recognized on the fly using a novel window based algorithm that employs machine learning (in particular Support Vector Machine) on data collected from inertial sensors. Also, simultaneously, information (e.g., heart rate variability) is extracted from vital signs (e.g., ECG) collected using bio-medical sensors. The recognized physical activity will be correlated with derived metrics from vital signs for preliminary diagnosis about a person’s health status. For example, switching from a state of rest to exertion should be accompanied by noticeable variation in heart rate. Otherwise, the person is deemed unhealthy.